Breaking the AI hegemony of the top manufacturers, domestic ASIC chips want to
01
Specialized and Refined, ASIC's Advantages Shine Through
Ever since AI large models began to become "smaller," integrating into mobile phones, PCs, and even self-driving cars, the ability of hardware devices to process these data models has become a new challenge. In other words, how can hardware keep up with the development of software? The NPU (Neural Processing Unit), a chip that was once somewhat redundant, has seen its status soar with the rapid advancement of AI applications.
Readers familiar with 3C products should know that the normal operation of a mobile phone is inseparable from the SoC (System-on-Chip, a system-level chip), which is an integrated circuit design that integrates multiple electronic components onto a single chip. Although the SoC is only as big as a fingernail, it is "fully equipped," with various modules integrated on it supporting the normal operation of all phone functions. For example, the CPU, which is responsible for the smooth switching of mobile applications, the GPU, which supports the rapid loading of game graphics, and the NPU, which is specifically responsible for AI computations and the operation of AI applications.
The CPU is the general-purpose "brain" of a computer, capable of processing various tasks, but it is neither specialized nor refined; the GPU is versatile and powerful, not only excelling in image processing but also becoming a must-have for everyone in the AI era due to its outstanding parallel computing capabilities. The NPU (Neural Processing Unit) can directly affect the strength of AI capabilities in 3C products because it mimics human neural networks and is specifically designed for executing machine learning algorithms. This specialized origin allows it to provide higher performance for AI tasks compared to GPUs.
Advertisement
This is the charm of ASIC (Application Specific Integrated Circuit) technology. ASIC chips are integrated circuit chips customized for specific purposes, and their characteristic is that the circuit logic is not programmable. Once designed and manufactured, they can only run one type of algorithm and perform one type of task. The most typical example is the mining chip.
High determinism is exchanged for high efficiency and performance optimization: since they are designed for specific applications, their circuits can also be highly optimized, thus minimizing power consumption to the greatest extent. In the field of AI applications, AI acceleration chips can be divided into three technological routes: GPU, FPGA, and ASIC, with the computational power of the chips increasing in that order.Back in reality, amidst the current AI wave, NVIDIA has already secured the top spot as the "seller of shovels" with its high-end GPUs, but it is not the only beneficiary. In previous articles, we mentioned that the veteran semiconductor leader Broadcom could "challenge" NVIDIA because it bet on AI software, a future technological benchmark, through acquisitions. However, more importantly, it is their design capabilities at the hardware level of computing power that has attracted many big customers who want to break NVIDIA's monopoly.
02
Design houses "lifted" to the forefront
Although NVIDIA's GPUs have taken all the limelight, many AI technology companies at home and abroad have not given up on developing their own chips.
The evaluation of self-developed chips by Amazon AWS may represent the voice of some AI companies: due to the increased use of AI deep learning, development teams are constrained by fixed computation limits, which in turn affects the development of their models and applications. "By investing in homemade chips, training costs can be reduced."
Amazon AWS's first-generation self-developed AI training chip, Trainium, not only saves 50% of training costs compared to other chips of the same level, but also significantly improves performance. The training chip Trainium 2, released at the end of last year, has increased its speed by 4 times compared to the first generation and can be deployed in computing clusters with up to 100,000 chips, greatly reducing the time to train models while improving energy efficiency by up to 2 times.
As these major companies begin to develop their own chips, the biggest beneficiaries are ASIC service providers, with Broadcom being a typical but not the only example.Broadcom, while valuing cloud-integrated software solutions, will invest $69 billion to acquire VMware, a leading global enterprise in virtualization and cloud computing infrastructure. However, AI software services can only be considered a visionary future bet, not an opportunity for Broadcom to leapfrog to become the AI chip manufacturer second only to NVIDIA — the real opportunity comes from its expertise in ASIC design services.
Developing AI training chips through ASIC technology is the most cost-effective method, which has been proven in the AI wave sparked by Alpha GO. Google's TPU (Tensor Processing Unit) is the most typical representative. In 2016, Google specifically created the TPU for deep learning, designed for its machine learning framework TensorFlow, and was used in AlphaGo. It has now evolved to the fourth generation, mostly deployed on the Google Cloud Platform, and some Tensor chips were integrated into Google's Pixel 9 series phones this year, handling AI computing tasks directly on local devices.
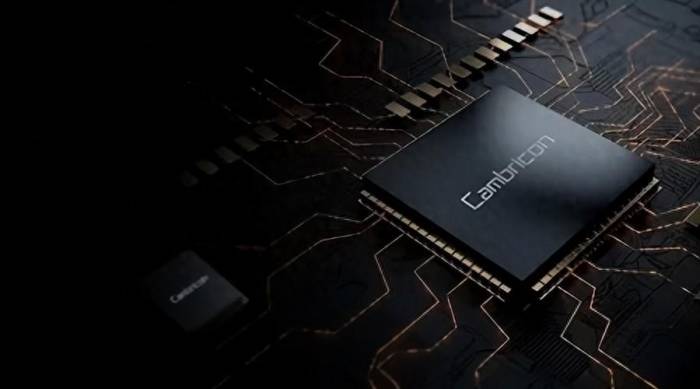
The design of Google's TPU chips is done by Broadcom. According to industry insiders, as a communication giant, Broadcom possesses a key tool for improving signal transmission efficiency — SerDes (Serializer/Deserializer) communication technology. It is worth mentioning that this technology was also acquired. In 2013, Broadcom acquired the SerDes division of LSI for $6.6 billion.
A brief introduction to LSI, which may be unfamiliar to younger readers: Founded in 1981, LSI Logic was already the largest ASIC enterprise in the 1990s and one of the pioneers in the ASIC industry. With a rich portfolio of IP core technologies, it had strong ASIC design and manufacturing capabilities, providing customers with high-performance, low-power, and complete ASIC solutions and products. The business model at the time was similar to today's, offering design services to companies that did not have the capability to design ASIC chips themselves; after the design scheme was approved, these companies would then place orders to purchase ASIC chips.
The SerDes communication technology helps ASIC chips by solving a key issue, namely chip-to-chip communication, which greatly appealed to Google.
An overseas hard technology investor stated last year that Google's ability to create large-scale clusters is very prominent, "NVIDIA's NVLink technology supports up to 256 GPUs for chip-level interconnection, while Google can connect 4096 TPU chips, which is 16 times that of NVIDIA." In early tests, the first-generation TPU's performance and power consumption far exceeded NVIDIA's GPUs of the same period. Therefore, when the battle of large models began and everyone was competing in computational power levels, Google also significantly increased TPU orders, pushing Broadcom to the forefront and bringing in two major clients, Meta and Microsoft.
Broadcom's own data indicates that its AI-related revenue for the fiscal year 2024 will increase from $3.8 billion to $7.5 billion. However, according to forecasts from several market institutions, just the Google TPU orders will bring in over $3 billion in revenue for Broadcom, and the overall revenue from AI chips will exceed $8 billion. Analysts at JPMorgan believe that for large-scale technology enterprises, AI custom chip solutions are an inevitable choice for the future, "but Broadcom is not necessarily the only one."The reason for delving deep into ASICs is that, under the "precise" chip restrictions by the United States, our country not only finds it difficult to import cutting-edge chips but also struggles to obtain the latest chip production tools, including design software. However, considering the difficulty of catching up with high-end GPUs, ASICs may present a good opportunity for AI companies in our country.
In the ASIC design service market, there are many participating manufacturers, including Broadcom, Marvell, MediaTek, Faraday Technology, Global Unichip Corp (GUC), and alchip, among others. However, there is no absolute leader in the global market, and domestic chip manufacturers such as Cambricon, Horizon Robotics, Insigma Technology, and Biren have advantages and opportunities to participate.
Taking Alibaba and Baidu as examples, both are procuring NVIDIA GPUs in large quantities while developing their own AI training chips, such as Alibaba's HanGuang chip and Baidu's Kunlun chip. This also confirms the judgment of industry insiders: in the short term, internet giants will use NVIDIA's existing products to catch up with OpenAI, but as the application scenarios for large models increase, cost-effectiveness will become an inevitable business consideration, benefiting the entire AI chip industry.
Under the urgent demand for domestic substitution, domestic manufacturers' deep neural network acceleration ASIC chips will also welcome favorable conditions. In 2017, Huawei released its first flagship smartphone chip, "Kirin 970," which was equipped with Cambricon's NPU module; in 2018, Huawei launched the "Kirin 980 chip," which also featured Cambricon's NPU.
However, meeting overall performance standards is not the only criterion. Generality, ease of use, and high performance are the three dimensions used by the industry to measure AI-specific chips. Due to the lack of a powerful software ecosystem similar to NVIDIA's CUDA, adapting to various AI algorithms has become a technical challenge, making it difficult for domestic chip manufacturers to compete with NVIDIA in terms of ease of use. Establishing their own supporting AI software ecosystem is a hurdle that domestic manufacturers will likely have to face.
Comment