Who is the best "special student"? From reading to writing, a horizontal test of
In the era of domestic AI large model competition for long text, Kimi has taken the lead in "breaking out of the circle" with its first-mover advantage. As industry giants like Baidu and Alibaba enter the field, the competition in the AI long text application track is becoming increasingly fierce.
01
Long Text Competition
The Productivity Tool Attribute of AI Large Models
"Long text, as the company's first step in 'landing on the moon,' is a new form of computer memory. It is very fundamental, and personalization is not achieved through fine-tuning; context defines the process of personalization." —— The speech by Yang Zhilin, founder of Moonshot AI (Kimi's parent company), has opened the prelude to the "long text" era of AI large models.
From thousands to hundreds of thousands of tokens, large models are becoming "visibly" longer at a "visible" speed. Compared to the 2 million word parameter volume of Moonshot AI's Kimi intelligent assistant, Baidu's Wenxin Yiyan has opened up long text processing functions of 2 to 5 million words, which is hundreds of times the previous highest document processing capability of 28,000 words; Alibaba's Tongyi Qianwen has announced an upgrade, opening up long text processing capabilities of up to 10 million words; 360's Zhiniao is currently in internal testing with 5 million words, and after the official upgrade, it will be integrated into the 360 AI browser.
Advertisement
"Competing" in long text has become the first milestone for basic general-purpose large models in the new season. What is the concept of 2 million words? Cao Xueqin's "Dream of the Red Chamber" has about 800,000 words, and J.R.R. Tolkien's "The Lord of the Rings" trilogy (including "The Fellowship of the Ring," "The Two Towers," and "The Return of the King") has a total of about 1.5 million words in Chinese, with 2 million words being slightly more than the total number of words in "The Lord of the Rings" trilogy.
Such long content can be read and summarized or summarized according to user needs in just a few seconds by large models with "long text" capabilities.Kimi has ignited the long text competition within large AI models.
Mainstream technology companies are so interested in the application of long text in large models largely because of the excellent monetization capability of the long text track. Long text models possess more accurate text understanding and generation capabilities, as well as stronger cross-domain transfer abilities. This is a very necessary capability support for creating industry experts in vertical fields, such as for some extensive medical literature, legal documents, financial reports, etc. Long text models have better understanding capabilities, correspondingly completing cross-domain learning and application, thus creating more professional medical assistants, legal assistants, and financial assistants, etc. This means that large AI models can have stronger productivity tool attributes.
02
True and false long text, numbers ≠ capabilities
When many large model companies announced breakthroughs in their products in the "long text" track in a very short time, some skeptical voices also emerged. Skeptics believe that what the later comers have launched is not true long text technology, but RAG technology. RAG is a technology known as retrieval-enhanced generation, which can search for relevant content from documents and provide this content to large models for reasoning.
The person in charge of the Dark Side of the Moon Company also emphasized to the media that unlike other companies' products, Kimi's long text is lossless compression technology for long contexts, while RAG is lossy compression technology. He gave an example, for instance, when reading a 1 million-word book, Kimi's long text technology will read every word and sentence one by one, and after reading 1 million words, it will summarize and analyze. RAG technology may only read the first line of each page of this book and then summarize and analyze. In terms of the final presentation effect, the content output by lossless compression technology is more real, comprehensive, and effective.
At present, large global models generally use the Transformer decoder as the core architecture. In order to achieve long context processing, researchers have made several improvements to the decoder architecture, mainly including the following four aspects—
First, adopt an efficient attention mechanism to reduce computational costs, enabling the processing of longer sequences during training, thereby increasing the sequence length during reasoning; second, implement long-term memory by designing explicit memory mechanisms to overcome the limitations of context memory; third, improve position encoding by optimizing existing encoding methods to achieve context extrapolation; fourth, process the context by additional pre-processing and post-processing means to ensure that the text input for each call to the large language model always meets the maximum length requirements.Long context, as a core technology, is not disclosed by various manufacturers. Currently, it is only possible to speculate on the long text technology of each company through other public channels. Taking the dark side of the moon as an example, its founder, Yang Zhi Lin, mainly discussed the implementation methods of long context in his academic papers Transformer-XL and XL-Net, with the former being an optimization of long-term memory and the latter being an optimization of special target functions. Baidu's ERNIE-DOC, on the other hand, has adopted optimization methods for both long-term memory and special target functions.
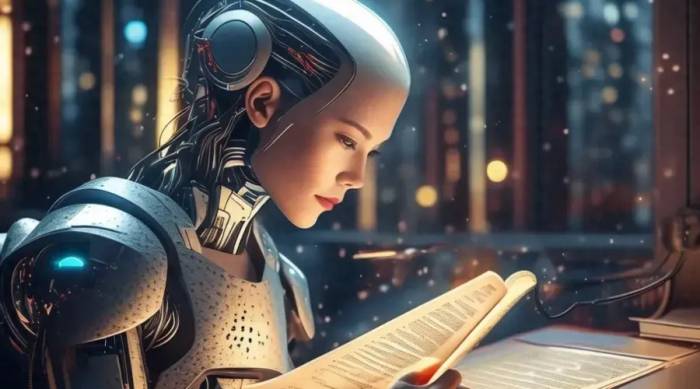
Alibaba's Qwen-7B has used an optimized position encoding algorithm called extended RoPE. Therefore, we speculate that domestic model manufacturers have been able to practice long context methods in a short period, possibly by iterating algorithms on the basis of existing accumulation, adopting a mix of multiple methods for optimization, and achieving rapid overtaking.
In fact, after a year of rapid iteration, the industry has long come to realize that the longer the text length is not necessarily better, and the effect is the foundation for AI large models to stand in the long text track.
03
The competition of four long text AI large models
After nearly a year of "involution," how is the performance of AI large models in the field of long text currently?
We selected four applications representing the first generation of long text large models, Kimi, representing long text support and focusing on Chat dialogue, Wenxin Yiyan (4.0 Turbo), Meta AI, which entered the long text application from the field of intelligent search, and "Orange Chapter," which is focused on the long text track, for a horizontal comparison, to show the current situation of AI large models in long text applications.In terms of testing methods, a horizontal comparison is made between the long-text applications of "reading" and "writing" to comprehensively showcase the current AI large model's long-text capabilities.
04
Reading Comprehension: Orange Chapter Stands Out
The reading comprehension test is divided into two parts: online and local file segments. The current part uses the instruction "Analyze the number of students admitted to Tsinghua University and Peking University in Chongqing through the college entrance examination in the past 10 years and present it in the form of a chart" to have Kimi, Wenxin Yiyan, Meta AI, and Orange Chapter read online materials while generating charts. This not only involves the AI large model's reading comprehension ability but also tests the current AI large model's partial multimodal capabilities through charts.
From top to bottom, and left to right, the results generated by Orange Chapter, Kimi, Wenxin Yiyan, and Meta AI are as follows:
The gap in the collection and organization of internet data by the four applications is very obvious. Kimi, without direct data available, only organized the number of students admitted by Tsinghua University in Chongqing in 2023 and 2016, and for Peking University, only the number of students admitted in 2023. "Orange Chapter," on the other hand, not only completed the comparison of the number of students admitted by the two universities in Chongqing over the past 10 years according to internet data as required, but also distinguished between the number of students admitted in physics and history for the years 2022 and 2023.
Meta AI, somewhat "straightforwardly," only organized the years for which it could directly collect data, with little effort in analysis or reasoning, which somewhat resembles the workplace attitude of "doing as much work as the salary dictates."
"Orange Chapter" can not only generate a clear table of data but also provides notes for users. By carefully reading the notes, we find that "Orange Chapter" clearly mentioned the three schools and various factors affecting the data when organizing and analyzing the data in 2020. The generation of such an answer means that "Orange Chapter" not only organized internet data but also analyzed and categorized it according to user requirements. At the same time, "Orange Chapter" also conducted a simple analysis of the data.In comparison, Wenxin Yiyan, which is also part of the larger Baidu ecosystem, exhibits a "science student's" caution in data processing. It is very prudent in estimating data, not only clearly marking "estimate, based on overall admission situation," but also using regional comparisons like "specific number of admissions in Chongqing is not detailed, but the total number in Beijing is relatively high" to enhance the accuracy of the data. Although it is difficult to directly extract table data, the analytical logic is clear, and it can be said to have "no credit but hard work."
In terms of local text reading, we selected a research report titled "C919 Volume Year, the Big Plane Takes Off with the Wind," which includes images, text, and table information, and asked four applications to read it. We used the command "Help me summarize these documents" to let the AI large model provide a summary.
Upon comparison, it was found that Kimi overlooked "C919's technical highlights and material applications" in the article summary, and "industrial chain company sorting" was also directly piled up in the expression. In contrast, "Orange Chapter" divided it into three categories: "airframe manufacturers," "material suppliers," and "aviation system suppliers," and then classified companies for each category. "Wenxin Yiyan" also provided a detailed list and summary of "localization rate and replacement process," which was more detailed in content summary. Unfortunately, Meta AI currently does not support local file uploads, which greatly weakens its application in reading comprehension.
From the perspective of summary content, "Orange Chapter" and "Wenxin Yiyan" are on par, but while "Orange Chapter" sorts out the content summary, it also attaches an "overall summary" at the end of the text. Its overall reading comprehension ability for long texts is more outstanding. With excellent online reading comprehension ability, "Orange Chapter" performed significantly better than the others in the "reading comprehension" test section.
05
Long Article Writing
Changing Content Generation Model
From content collection, organization to creation, it is more valuable to let AI generate content that can be transformed into a travel brochure or guidebook with the command "Help me write a long article with the theme: Introducing the top ten museums in Beijing," rather than asking AI to generate a readable article based on the college entrance examination essay to elaborate on life and values.Upon receiving the command, four AI large models demonstrated distinctly different processes and methods in content generation. Among them, Kimi and Wenxin Yayan directly composed an "article" similar to a collection of search results for us. Kimi and Wenxin Yayan generated lengthy texts based on the instructions, which included introductions to 10 major museums in Beijing. There was no deviation from understanding to answering the question. However, facing the same instructions, "Orange Chapter" first generated an outline for the article, which users can directly modify and adjust within the outline.
Before generating a lengthy article, Orange Chapter first generates an adjustable article outline. After the user confirms that the outline generated by "Orange Chapter" is correct, they can click the "Generate Full Text" button (if they are particularly dissatisfied, they can even directly click "Change Outline"). Based on the outline, "Orange Chapter" completed a 13,158-word long article, which not only provides detailed introductions to 10 Beijing museums but also offers suggestions for visiting and touring. At the end of the article, there are references.
The final result generated by Orange Chapter is quite excellent in both word count and article structure.
After receiving the command, Meta AI directly listed the information of "Top Ten Museums in Beijing" and also prompted users to use Meta's "Writing Cat AI" to complete the article generation.
Meta AI will have a clear "Writing Cat AI" prompt on the results interface.After selecting to enter the "Writing Cat AI" interface, you will see an interface similar to an online light office. Here, not only will the search content of Meta AI be reorganized, but there will also be two prompts at the bottom: "Write Content" and "Write Outline." After selecting "Write Outline," Meta's "Writing Cat AI" will also create an article outline based on the search content just now.
Writing Cat AI completes the creation of the article based on the search content of Meta AI. In the Writing Cat AI interface, we can not only adjust the details such as the font interface but also input commands to insert or rewrite, integrating the light office application with AI. However, looking at the content generated by default, Meta's "Writing Cat AI" seems to have less depth in the article completed for this command than the Orange Chapter.
However, from the steps of long text completion and the presentation of the work, both Meta AI and the Orange Chapter are no longer simply generating long text content in a dialogue manner. From the analysis and understanding of the command to the generation of the long text outline and the complete generation of the content, the long text generation process of these two AI large models has become similar to that of a real person. At the same time, whether it is Meta's "Writing Cat AI" or the built-in Word editor of the Orange Chapter itself, they have integrated AI large models with light office, which means that AI long text office has a one-stop office prototype.
06
One-stop Office: The Duel of Orange Chapter and Meta AI
Integrating AI large models with light office platforms, the Orange Chapter and Meta AI have shown us a lot of new ideas in the application of long text. It should be noted that Meta AI currently uses the "Writing Cat AI" under Meta to combine light office with Meta AI's long text capabilities. Although it has achieved "interconnection" within the software, it is still two completely independent AI applications, and there is still room for improvement in terms of user consistency. In terms of specific AI + light office design ideas, the Orange Chapter and Meta AI actually have significant differences.
While embedding the "Intelligent Assistant," the Orange Chapter has a clear tool attribute in its functions, emphasizing applications such as "full text correction" and "format arrangement." In addition to adjusting fonts and paragraphs in the main interface, the expanded functions are basically placed on the right side of the interface.The "Orange Section" is more text-function oriented in its functional design.
In contrast to the "Orange Section's" "focus" on text processing, the "Mita Writing Cat AI" pays more attention to the overall integration of AI functions. Its central operation interface is divided into "Start," "Efficiency," and "Review" sections. Users can not only directly adjust the font and paragraphs of the article content on the "Start" interface but also enable AI to help achieve "full-text rewriting," "full-text summarization," "intelligent typesetting," and other functions on the "Efficiency" interface. At the same time, by clicking the "Collaboration" button in the upper right corner of the "Writing Cat AI" content interface, users can invite others to collaborate or directly publish their creative content, which is already moving closer to the design of light text office tools like Tencent Docs and Shunwei Docs.
As a relatively opposing existence, Mita may itself want to build the "Writing Cat AI" into an independent AI writing platform. When users click on the icon next to the "Collaboration" in the upper right corner of the operation interface, the entire left side of the interface will display different AI tool suites according to the five menus of "AI Writing," "Proofreading," "Images," "Dictionary," and "Comments."
Mita's "Writing Cat AI" showcases various AI tools in a platformized manner.
Here, we focused on trying the "Proofreading" section of the "Writing Cat AI," as WPS has already categorized the "Document Proofreading" function into the membership area. Such AI platforms can directly and accurately proofread long text content, which undoubtedly has considerable practicality.
The "Proofreading" function of the "Writing Cat AI" is divided into "Content Suggestions," "Fact-checking," and "Full-text Summary" sections. This is somewhat different from our understanding of the "proofreading" function, as it adds "Fact-checking" and "Full-text Summary" to the traditional word proofreading, with these two functions being more focused on examining the content of the article.
The "Proofreading" function of the "Writing Cat AI" has a certain degree of innovation.
In comparison, the "Orange Section" is more down-to-earth in its "Proofreading" function. The "Full-text Correction" function of the "Orange Section" is directly divided into "Error Correction," "Readability," and "Full-text Suggestions" sections. "Error Correction" mainly targets word and phrase errors, while "Readability" is aimed at optimizing article sentences. Users can choose to "Ignore" or "Adopt" suggestions, and the design of the left and right sidebars makes operation very convenient.Orange's proofreading feature is more in line with everyday office experience.
The concept of "AI+light office" is not entirely new, as Tencent Documents and Quark Smart Documents are also actively integrating AI large models to enhance the user experience. Orange and Meta AI, on the other hand, integrate from the perspective of AI large models into the light office platform. For now, these two approaches do not conflict. Orange and Meta AI often complete the closed loop of generative AI content through text editing, which is equivalent to reading and understanding internet content to long text generation and editing. AI large models can meet user needs in a one-stop manner.
Whether it's self-media practitioners, journalists, or white-collar and student groups with article writing needs, products like Orange and Meta AI can undoubtedly effectively improve learning and office efficiency.
07
In conclusion: The rise of AI's niche application track
For chat-like large models to gain users in the C-end mass market, there are essentially two paths: one is to be a productivity tool, and the other is to be an entertainment tool. Since Kimi has driven AI large models to "in-roll" in the long-text track, AI large models that can reflect productivity value are more in line with current terminal consumer market demands.
From content creation to professional fields such as law and finance, AI large models with long-text capabilities can quickly extract, organize, and even analyze information, acting as an "assistant" role. While reducing the workload for users, they also implement the value of AI tool deployment.
Even as an entertainment tool, long text can provide more context and detail information to assist the model in judging semantics, further reducing ambiguity. Moreover, induction and reasoning based on the provided facts are more accurate. This means that Agents (intelligence entities) that focus on "emotional companionship" can have long-term "memory," thereby providing users with a coherent interactive experience and promoting the rise of the entire AI application.
Comment